
Empirical calibration of confidence intervals was most effective when adjusting for the unmeasured confounding bias.

ResultsĮmpirical calibration increased coverage of the 95% confidence interval of the treatment effect estimate under most bias scenarios but was inconsistent in adjusting the bias in the treatment effect estimate. The performance of the empirical calibration was evaluated by determining the change in the coverage of the confidence interval and the bias in the treatment effect estimate. The simulations consisted of binary treatment and binary outcome, with biases resulting from unmeasured confounder, model misspecification, measurement error, and lack of positivity. The effect of empirical calibration of confidence intervals was analyzed using simulated datasets with known treatment effects. Although empirical calibration has been used in several large observational studies, there is no systematic examination of its effect under different bias scenarios. An extension of this technique calibrates the coverage of the 95% confidence interval of a treatment effect estimate by using negative control outcomes as well as positive control outcomes, which are outcomes for which the treatment of interest has known effects.
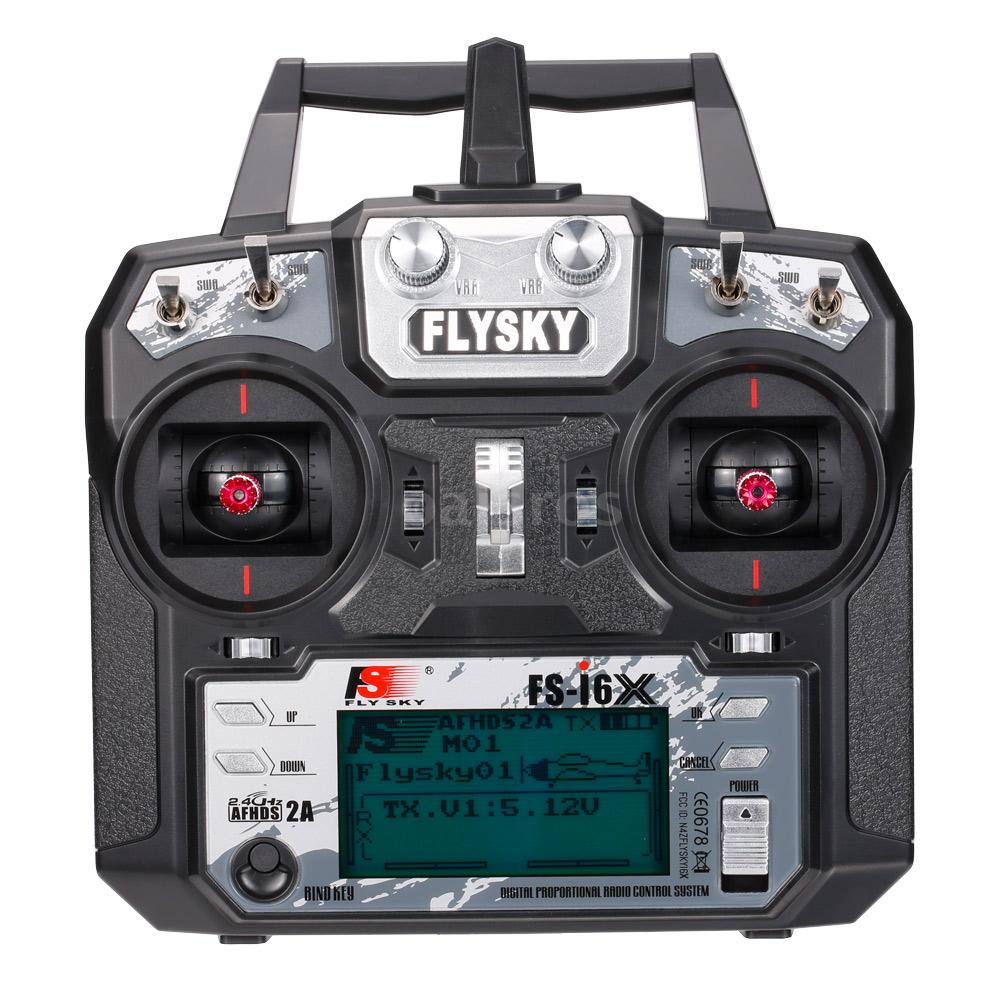

The empirical calibration procedure is a technique that uses negative control outcomes to calibrate p-values. One method for adjusting for the residual biases in the estimation of treatment effects is through the use of negative control outcomes, which are outcomes not believed to be affected by the treatment of interest. Estimations of causal effects from observational data are subject to various sources of bias.
